Note: Claims that ECT is safe and effective are common in electroconvulsive therapy study introductions. These are assertions without a scientific basis. See Electroconvulsive Therapy for Depression: A Review of the Quality of ECT versus Sham ECT Trials and Meta-Analyses and System_IV_Instruction_Manual_Rev22.pdf page 7, section 1
Whole-Brain Functional Connectivity Dynamics Associated With Electroconvulsive Therapy Treatment Response
ZeningFua JingSuiaghRandallEspinozad KatherineNarrdShileQiaMohammad S.E.SendiacChristopher C.AbbotteVince D.Calhounabcf
aTri-Institutional Center for Translational Research in Neuroimaging and Data Science (TReNDS), Georgia State University, Georgia Institute of Technology, Emory University, Atlanta, GeorgiabDepartment of Psychology, Computer Science, Neuroscience Institute, and Physics, Georgia State University, Atlanta, GeorgiacDepartment of Electrical and Computer Engineering, Georgia Institute of Technology, Atlanta, GeorgiadDepartments of Neurology, Psychiatry and Biobehavioral Sciences, University of California, Los Angeles, Los Angeles, CaliforniaeDepartment of Psychiatry, University of New Mexico, Albuquerque, New MexicofDepartment of Psychiatry, Yale School of Medicine, Yale University, New Haven, ConnecticutgNational Laboratory of Pattern Recognition, Institute of Automation, Chinese Academy of Sciences, Beijing, ChinahUniversity of Chinese Academy of Sciences, Beijing, China
https://doi.org/10.1016/j.bpsc.2021.07.004
Under a Creative Commons license
Referred to by
Fabio Sambataro, Robert Christian Wolf Time After Time: Electroconvulsive Therapy Modulates the Brain’s Functional Network Connectivity Dynamics
Biological Psychiatry: Cognitive Neuroscience and Neuroimaging, Volume 7, Issue 3, March 2022, Pages 243-245Purchase PDF
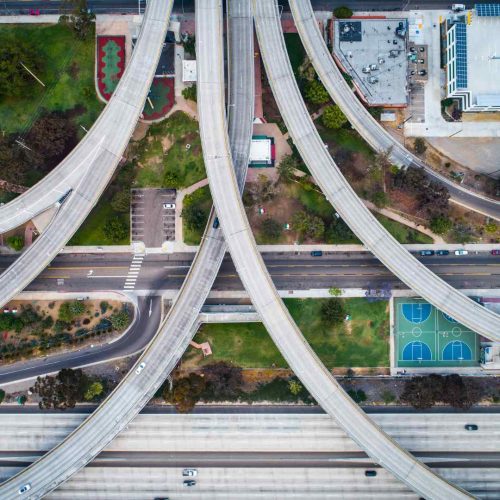
Abstract
Background
Depressive episodes (DEPs), characterized by abnormalities in cognitive functions and mood, are a leading cause of disability. Electroconvulsive therapy (ECT), which involves a brief electrical stimulation of the anesthetized brain, is one of the most effective treatments used in patients with DEP due to its rapid efficacy.
Methods
In this work, we investigated how dynamic brain functional connectivity responds to ECT and whether the dynamic responses are associated with treatment outcomes and side effects in patients. We applied a fully automated independent component analysis–based pipeline to 110 patients with DEP (including diagnosis of unipolar depression or bipolar depression) and 60 healthy control subjects. The dynamic functional connectivity was analyzed by a combination of the sliding window approach and clustering analysis.
Results
Five recurring connectivity states were identified, and patients with DEPs had fewer occurrences in one brain state (state 1) with strong positive and negative connectivity. Patients with DEP changed the occupancy of two states (states 3 and 4) after ECT, resulting in significantly different occurrences of one additional state (state 3) compared with healthy control subjects. We further found that patients with DEP had diminished global metastate dynamism, two of which recovered to normal after ECT. The changes in dynamic connectivity characteristics were associated with the changes in memory recall and Hamilton Depression Rating Scale of DEP after ECT.
Conclusions
These converging results extend current findings on subcortical-cortical dysfunction and dysrhythmia in DEP and demonstrate that ECT might cause remodeling of brain functional dynamics that enhance the neuroplasticity of the diseased brain.
Keywords
Clustering analysis
Depressive episodes
Dynamic functional connectivity
Electroconvulsive therapy
Metastate dynamism
Neuroplasticity
Depression is one of the leading causes of disability globally, affecting millions of lives and being the second contributor to the global burden of disease (1,2). Fortunately, depression is among the most treatable of mental disorders, with many forms of treatments successfully applied in clinical trials. Electroconvulsive therapy (ECT) under general anesthesia and with the use of adequate muscle relaxation is regarded as a potent treatment with rapid acting in severe mental illness (3, 4, 5), with 48% to 65% of the patients with depression recovered with ECT as compared with 10% to 40% with chemical antidepressant treatments (5, 6, 7). However, the relationship between brain changes and ECT response is still far from understood. Characterizing ECT responsive brain markers and unveiling the neurobiological underpinnings of ECT using less invasive approaches will help to improve the treatment of severe depression with a more benign side effect profile (3,8,9).
Despite its efficacy, the side effects of ECT, especially the extent of short-term and long-term cognitive deficits, can be substantial (10,11). Multiple lines of evidence have shown that ECT causes extensive memory impairments, including anterograde amnesia, retrograde amnesia, and memory complaints (12, 13, 14). The research regarding memory changes is somehow inconsistent, with either impaired memory function (15,16) or improved memory function reported (17,18), making the persistence, severity, and precise characterization of such memory changes still a debated topic in this area. Although many treatment modifications have been introduced, including new electrode placements and waveforms (14,19, 20, 21), which have provided a better overall cognitive profile after ECT, those side effects, in particular the short-term memory changes, have not been eradicated.
The human brain continuously integrates information among multiple brain regions related to the neural basis of perception, cognition, and emotion (22,23). The changes in such integration (so-called functional connectivity [FC]) play a critical role in the generation of mood symptoms in patients with depression. Abnormal FC during both cognitive and emotional tasks has been found in patients with depression, which provides insights into how the dysfunctional brain relates to abnormal behavioral response patterns induced by depressive episodes (DEPs) (24, 25, 26). The FC change is also an important therapeutic target in the treatment of depression (27, 28, 29). In addition, the alterations in brain FC are reliable indicators of memory changes associated with age (30,31), brain diseases (32,33), and task events (34). Taken together, FC might serve as a key intersecting point that can help to link DEP, treatment outcomes, and treatment side effects. However, conventional ECT studies assumed constant FC during the resting state, and constant FC might unfortunately oversimplify the functional relationships between brain regions (35,36). The human brain is highly dynamic and incorporates the evolution of local activities and the reconfiguration of brain interactions, adapting to both internal and external stimuli (37,38). Challenging the static assumption of FC, recent studies have shown that functional magnetic resonance imaging FC continuously fluctuates in different temporal scales (35,36,39,40), and such time-varying characteristics reflect neural mechanisms in cognitions and diseases that cannot be captured by static analysis (41, 42, 43, 44).
To date, no studies have examined dynamic FC (dFC) in the context of ECT response in patients with DEPs. In this work, we aimed to comprehensively investigate different dFC characteristics in DEP and explore the dFC changes responsive to ECT. We hypothesized that DEP might be associated with abnormal occurrences in dynamic brain states and abnormal whole-brain dynamism, such as dynamic FC fluidity and range. We also hypothesized that ECT would provoke alterations in the occurrence of brain states and global dynamism, which might be associated with changes in depressive symptoms and memories. We applied an analytic framework combining a novel independent component analysis–based pipeline, the sliding window cross-correlation, and two clustering analyses to a large sample dataset including 110 patients with DEPs and 60 healthy subjects. This framework is a powerful approach that can probe dFC characteristics from different perspectives (36,45), robust against variation in data quality, analysis, grouping, and decomposition methods (46).
Methods and Materials
Participants
Healthy control (HC) participants and patients with DEP (including diagnosis of unipolar or bipolar depression) were recruited from the University of New Mexico and the University of California, Los Angeles. Written informed consent was obtained from all participants under protocols approved by the Institutional Review Boards of University of New Mexico and University of California, Los Angeles. We selected data samples according to the following criteria: 1) subjects without neurodegenerative or neurologic disorders; 2) subjects without other psychiatric disorders, such as schizophrenia; 3) subjects without current alcohol or drug dependence, without pregnancy; 4) subjects with head motion ≤ 3° rotation and ≤ 3-mm translation; and 5) subjects with good functional data normalization (45,47). These criteria yielded a total of 110 patients with DEP and 60 HC subjects. Patients were scanned within 7 days of the ECT start and of finishing the ECT series. HC subjects were scanned twice between 4- and 6-week intervals. Details of demographics, clinical assessments, and the ECT protocol are provided in Table 1 and the Supplement.
Table 1. Demographics and Clinical Characteristics of Participants
Characteristics | Participants | t Statistic, p Value | |
---|---|---|---|
DEP (n = 110) | HC (n = 60) | ||
Age, Years, Mean ± SD | 56.08 ± 15.76 | 48.57 ± 14.91 | .0029 |
Gender, Female/Male, n | 68/42 | 34/26 | .5152 |
Handedness, Right/Left, n | 103/7 | 56/4 | .8725 |
Bipolar/Unipolar, n | 6/104 | NA | NA |
Psychotic/Nonpsychotic, n | 28/82 | NA | NA |
RUL/Mixed RUL-Bitemporal, n | 75/35 | NA | NA |
Number of ECT, Mean ± SD | 11.20 ± 3.21 | NA | NA |
Responder, n (%) | 64 (58%) | NA | NA |
Remitter, n (%) | 42 (38%) | NA | NA |
HDRS (Pre-ECT), Mean ± SD | 25.69 ± 6.18 | NA | NA |
HDRS (Post-ECT), Mean ± SD | 11.46 ± 8.76 | NA | NA |
Memory Recall (Pre-ECT), Mean ± SD | 74.54 ± 63.34 | NA | NA |
Memory Recall (Post-ECT), Mean ± SD | 68.16 ± 29.95 | NA | NA |
DEP, depressive episode; ECT, electroconvulsive therapy; HC, healthy control; HDRS, Hamilton Depression Rating Scale; NA, not applicable; RUL, right unilateral.
Flowchart of Dynamic FC Analysis
Before the dFC analysis, we performed typical functional magnetic resonance imaging preprocessing based on SPM12 within the MATLAB release 2016a (The MathWorks, Inc.) environment (detailed procedures are provided in the Supplement). Flowchart of the dFC analysis is displayed in Figure S1. The Neuromark pipeline (48) was first used to calculate highly corresponding component templates. The templates were used as the references to guide the back-reconstruction of independent components for each subject from the DEP dataset. Detailed information of the Neuromark is provided in the Supplement. A sliding window approach was used to estimate dFC. Then, subject exemplars were chosen as those windows with local maxima in FC variance, and k-means clustering was performed on the subject exemplars. Finally, the hard-clustering analysis and the fuzzy metastate analysis were conducted on all dFC estimates.
Dynamic FC Estimation and Clustering Analyses
We used a tapered window, created by convolving a rectangle (width = 80, repetition times = 36.8 s) with a Gaussian (σ = 3 repetition times) for segmenting the time courses. The window size within the range of 30 seconds to 1 minute can capture reliable resting-state FC dynamics (35). We estimated covariance from the regularized precision matrix, which is obtained via the graphical lasso method on the windowed data. The covariance matrices of windows were finally concatenated to form a C × C × W (C is the number of components and W is the number of windows) array for each subject that represents the dynamic changes in FC as a function of time.
A hard-clustering analysis based on the k-means clustering was first applied to the windowed dFC estimates. The fractional rate (FRA) and mean dwell time (MDT) (36,47) were calculated based on the clustering vectors, evaluating how frequently and how long an individual stays in a given state. A fuzzy metastate analysis based on the k-means clustering was further performed to investigate the global characteristics of the whole-brain dFC (49). Four dynamism measures were defined by this metastate analysis, including 1) metastates number, 2) metastates switching times, 3) occupied metastates range, and 4) overall traveled distance. Details of the clustering analyses can be found in the Supplement.
We employed a general linear model to investigate whether patients with DEP and HC subjects have different FRA and MDT in each functional state and whether they have different dynamism measures within pre- and post-ECT sessions, respectively. Age, sex, and site were used as the covariates in the statistical analysis. A pairwise t test was then performed to investigate whether the dFC characteristics (FRA, MDT, and 4 dynamism measures) are responsive to ECT by comparing them between pre-ECT DEP and post-ECT DEP. We also performed a pairwise t test between 2 HC sessions to examine whether the dFC characteristics change between HC scans. To further investigate whether the changes in dynamic characteristics are associated with the changes in symptoms and cognitive performance after ECT, we calculated the difference in dynamic features and the difference in Hamilton Depression Rating Scale-17 items and memory recall scores between pre- and post-ECT session and used the general linear model to examine their potential associations. For comparison, we further calculated the Pearson correlation to measure the static FC between components and performed the same statistical analysis on the static FC estimates (results are provided in the Supplement).
Results
Brain Parcellation
A total of 53 pairs of components were identified by the Neuromark pipeline (Figures S2 and S3), arranging into 7 functional networks, including the subcortical (SC) (5 components), auditory (AUD) (2 components), sensorimotor (SM) (9 components), visual (VIS) (9 components), cognitive control (17 components), default mode (DM) (7 components), and cerebellar (4 components) networks.
Group Difference in Occurrences of dFC States
Five dFC states were identified by k-means clustering, and the results are displayed in Figure 1 (detailed methodologies for clustering brain states are provided in the Supplement). The upper panel shows the FC matrix representing the centroid of a cluster and reflecting a dynamic brain state stably present within this dataset. The middle panel shows the functional profile of each brain state that retains only strong connectivity (absolute FC > 0.2). The lower panel shows the histogram of connectivity strength of FC pairs for each brain state. We note that brain states presented many distinct FC patterns and showed different connectivity distributions validating our hypothesis. State 4 accounted for more than 40% of all windows and was the most sparsely connected brain state with weak interregional connectivity. FC patterns in the other four states were observed less frequently (occurrences are between 11% and 17%) but represented connectivity diverging substantially from each other. State 1 was the most interconnected brain state, with strongly positive connectivity within sensory networks and antagonism between SC and sensory networks. A large sensory module was present, composed of components dedicated to the AUD, SM, and VIS networks. States 2, 3, and 5 displayed different within-network connectivity and between-network antagonism. State 2 revealed strongly functional integration between posterior DM components and anterior DM components. In state 3, consistently positive FC was observed between SC and sensory networks. Components from SC and sensory networks also acted in synchrony with the hippocampus component that was assigned to the cognitive control network. Brain states can also be differentiated by the FC between sensory networks (i.e., FC between AUD and SM, between AUD and VIS, and between SM and VIS). In most dynamic states, the FC between sensory networks showed at least weak positive correlations. However, in state 5, components from different sensory networks showed negative correlations, indicating functional segregation of the sensory module.

The results of group comparisons are shown in Figure 2. Compared with HC subjects, pre-ECT patients with DEP had significantly lower FRA and shorter MDT in state 1 (p < .05, false discovery rate [FDR] corrected). After ECT treatment, patients with DEP showed similar abnormalities in state 1. Beside decreased FRA and MDT in state 1, post-ECT patients with DEP had increased FRA in state 3 compared with HC subjects (p < .05, FDR corrected).

Group Difference in Higher Dimensional Metastate Dynamism
According to the results in Figure 3, compared with HC subjects, patients with DEP passed through fewer distinct metastates and stayed in a smaller radius of the metastate space (p < .05, FDR corrected). Patients with DEP also traveled less frequently between occupied metastates and less overall distance (p < .05, FDR corrected). After ECT treatment, two dynamism measures recovered to normal without showing any difference between patients and HC subjects. Post-ECT patients with DEP still showed the lower metastate number and metastate space span, although the differences were smaller (p < .05, FDR corrected).

ECT Responsive Dynamic Characteristics
Two brain states were identified with longitudinal discriminating patterns between pre-ECT DEP and post-ECT DEP (Figure 4). The FRA and MDT of state 3 significantly increased in patients with DEP after ECT treatment (p < .05, FDR corrected). In contrast, the FRA and MDT of state 4 decreased in patients with DEP after ECT (p < .05). For comparison, we also examined the changes in occurrences of brain states between two HC sessions, and there is no significant change in FRA and MDT in HC subjects (p > .05). Figure 5 displays the results of the ECT responsive metastate dynamism. All metastate measures significantly discriminated pre- and post-ECT DEP (p < .05, FDR corrected), with similar increasing trends after ECT treatment. Still, there is no difference identified in metastate measures between two HC sessions.


Association With Depressive Symptom Scores and Memory Recall
Whether the captured dynamic characteristics of brain FC can index the changes in depressive symptoms and memory function during ECT was further investigated. We did not observe significant correlations between changes in antidepressant response/memory performance and those dynamic characteristics across all subjects with DEP. However, we found interesting associations when we divided the subjects into ECT responder/nonresponder and memory decline/rise subgroups.
For ECT responders, reduced Hamilton Depression Rating Scale was positively correlated with changes in the MDT of state 1 (r = 0.4001; p = .0012, FDR corrected) (Figure 6). This positive correlation was still significant if we restricted the analysis to ECT remitters (r = 0.3902, p = .0117). We also found that the memory decline (percent recall of the Hopkins Verbal Learning Test-Revised and Repeatable Battery for the Assessment of Neuropsychological Status decreased after ECT) was positively correlated with decreases in FRA and MDT of state 4 (FRA: r = 0.4540, p = .0013, FDR corrected; MDT: r = 0.3280, p = .0244) (Figure 7). In contrast, memory decline was negatively correlated with increases in the metastate number and metastate space span (metastate number: r = −0.3182, p = .0293; metastate space: r = −0.3036, p = .0380). In contrast, memory rise (percent recall of the Hopkins Verbal Learning Test-Revised and BRANS increased after ECT) was positively correlated with increases in the metastate changes and total distance (metastate change: r = 0.3170, p = .0338; metastate total distance r = 0.3132, p = .0362).


Discussion
In this work, we investigated dynamic FC in a resting-state dataset with pre- and post-ECT sessions and found that patients with DEPs have significantly different occurrences in the dynamic states and a limited dynamic range and less dynamic fluidity compared with HC subjects. More interestingly, the above-captured dynamic characteristics changed significantly following ECT treatment, and the changes were associated with alterations in memory recall and depressive symptoms after ECT.
Abnormalities in Dynamic State Occurrences and Global Dynamism of Whole-Brain dFC
Increasing evidence is provided for supporting that the dynamic information embedded in the brain can be well-captured by the sliding window approach even using a small number of time points (35,50). The recurring FC state, a short period during which FC topography remains quasi-stable in a conceptual analogy to electroencephalography microstate, is a widely accepted representation of dFC (36). In this study, by combining the sliding window dFC and k-means clustering, we identified 5 brain states that recur in both patients and control subjects. The identified brain states 1, 2, and 3 have synchronous patterns between regions within the sensory networks but with significant transitions of the synchronization between subcortical and sensory networks. A previous study has observed frequently bidirectional transitions between striatal and somatosensory networks in rats (51). Our results extend this finding by showing that the subcortical network also has significant transitions with cortical networks in the human brain and provide further evidence indicating the presence of both bottom-up and top-down processing involving high-order cortical and low-order subcortical regions at rest (52,53). State 4, the most frequently occurring brain state, has the sparsely connected FC patterns that resemble static FC. Such a brain state with weak and diffused FC has been widely captured in previous studies, typically accounting for the largest percentage of time (43,45,54,55). It is speculated that this state represents the average of those additional brain states that are less variable to be separated (36).
Patients with DEPs tended to spend consistently less time in state 1, a brain state characterized by the most strong FC (both positive and negative) between brain regions, in both pre- and post-ECT sessions. This result is in line with previous findings of hypoconnectivity in depressive disorder (56,57) and further provides a potential explanation of reduced connectivity between frontoparietal control systems and networks involved in internal or external attention. Fewer occurrences in the brain state with strong prefrontal-subcortical connections might influence the information-transferred efficiency in the prefrontal-subcortical pathways, which further results in more efforts spent by patients to reappraise negative stimuli characterized by accentuated activation in subcortical regions (58,59).
We also found that patients have smaller metastate dynamism than HC subjects, indicating a reduced functional brain fluidity and limited dynamic range in DEPs. These metastate results are consistent with our dFC state results described above, because it is believed that less time across well-defined dFC states with strong connectivity profiles can result in decreased diversity in visited metastates (60). The identified abnormal global dynamism is also supported by previous findings that pairwise brain FC showed higher temporal stability in patients with major depressive disorder (61,62). It is believed that the reduced irregularity of resting-state FC is associated with network dysfunction in depression (63). The stable pairwise FC might reflect self-focused thinking, because the systems involved in this FC are believed to support autobiographical memory or prospection (64). Our results extend previous findings by showing that not only the pairwise FC between certain brain systems but also the global functional brain network exhibit less variability in patients with depression.
ECT Responsive State Occurrences and Global Dynamism
We observed that the occurrences of dFC states are responsive to ECT treatment. On one hand, the occurrence of state 3 increased after ECT treatment. State 3 has consistently negative connectivity between the thalamus and DM network components. The thalamus is one of the most important brain regions that have been subjected to intense scrutiny in DEPs. Increased thalamic metabolism (65,66) and increased thalamic connectivity (67) to the DM network in DEPs have been widely observed in previous studies, suggesting that activity in the thalamus is excessively coupled with the activity in the DM network in subjects with depression. More occurrences in dFC state 3 with negative thalamus-DM connectivity will decrease the FC between the thalamus and DM network, which can be a potential mechanism of ECT for normalizing the hyperactivity and hyperconnectivity in DEPs. State 3 also shows positive connectivity between the hippocampus and SC/sensory networks. The disrupted function and structure in the hippocampus have become a particular area of interest in DEPs over decades (28,68). A wide range of hippocampus changes has also been linked to ECT response, especially the increased hippocampal connectivity (28) and enlargement of hippocampal volumes (69). In light of these findings, we are encouraged by the findings of increased occurrence in state 3 after ECT. We speculate that the increased hippocampal volumes induced by ECT allow for the brain to have more capacity to stay in a brain state with a large amount of communication between the hippocampus and cortical regions, which further results in enhanced hippocampal connectivity at an average sense.
In contrast, decreased occurrence of dFC state 4 was identified after ECT treatment. State 4 is the most commonly identified dynamic state, with weak and diffuse connectivity patterns. A previous study of dFC in children revealed that the occurrence of such a weak connectivity state is related to the content of self-focused thought (70). Depression is associated with an increased tendency to be more self-focused (71,72), and the modification of self-focus is believed to be important for the treatment of depression (73). Taken together, our result reveals that ECT might normalize the activity of self-focusing by decreasing the occurrence into a brain state that is involved in self-referential processing.
Another interesting finding is that metastate dynamism is responsive to ECT with an increasing trend after ECT. This result provides further evidence of the potential relationships between dynamic fluidity and dFC state occurrences. Specifically, ECT may normalize the dynamic range and fluidity of the brain by decreasing the time that DEPs stay trapped in poorly defined dFC states, where the dynamic interplay between regions is less marked (60). Prominent theories of ECT response have highlighted the disconnections of FC between brain systems in treatment-resistant depression, which can be normalized by ECT treatment (74). Indeed, resting-state FC is regarded as an important predictor of treatment response to ECT in the literature (28,74,75). However, findings in this field are inconsistent and even contradictory to some extent, with both increased DM FC (74, 75, 76) and decreased DM FC identified following the treatment (77,78). Although such inconsistency may be partially due to the inherent heterogeneity of depression (79), we argue that metastate dynamism can provide a potential explanation of these disparities. Previous imaging investigations have indicated ECT to be a potent stimulator of neuroplasticity of the human brain (80). We speculate that ECT normalizes the neuroplasticity of the brain, reflecting by increasing dynamic range and fluidity rather than regulating FC directly. The enhanced neuroplasticity would allow the diseased brain a better ability to change and reorganize its FC for purpose of adapting to different situations.
Associations Between Dynamic Characteristics and Treatment Outcomes and Side Effects
For ECT responders and ECT remitters, we found that the change in the dwell time of state 1 is correlated with therapeutic outcomes. This finding indicates that the change in the continuous staying time of a state with the strongest dFC patterns might play a mechanistic role in the efficacy of ECT treatment for DEPs. We also found that the decreased occurrence of state 4 induced by ECT is correlated with memory recall decline in DEPs after ECT treatment. As discussed earlier, state 4 corresponds with the content of self-focus, and decreased occurrence in this state might reflect a reduction of self-focus in DEPs. Previous research has shown that high levels of ruminative analytic thinking in depression might be critical for the maintenance of overgeneral memory, and reducing self-focus will reduce autobiographical memory (81). In contrast, ECT has long been thought to exert significant effects on autobiographical memory (82,83). Our result might build a bridge between the decline in memory recall and self-focus from a dynamic perspective. Specifically, ECT will alleviate ruminative self-focus in patients by reducing their time spent in the self-focused state, which therefore exerts an adverse effect on memory recall (84).
We further show that memory recall decline after ECT is significantly correlated with increased dynamic range measured by metastate number and span. These findings are in line with a previous observation of negative associations between the variability of dFC and cognitive performance (85). Similarly, increased variance of FC was identified to be associated with slower reaction times on an attention task (86), and increased FC dynamics are linked to misses more often than hits in a perceptual attention task (87). Based on this and other prior work, we posit that the dynamic range of brain FC might be related to the degree of mind wandering, and ECT would increase the dynamic range. This in turn facilitates mind wandering during the resting state, leading to the more responsive time of the brain once memory recall is demanded (85). As opposed to dynamic range, we also found that increased dynamic fluidity is associated with memory rise after ECT. The metastate changes and distance reflect the flexibility of the brain to shift between different network configurations during different tasks and rest conditions. Studies have shown that such flexibility might index the varying degrees of cognitive impairment related to brain diseases (88,89), and the more flexible FC might be a correlate of more vivid and specific memory recall (89). We argue that although ECT increased both dynamic range and fluidity in DEPs, these dynamic characteristics could be different indicators of memory decline and rise, underlying different neural mechanisms that are related to ECT.
Acknowledgments and Disclosures
This work was supported by the National Institutes of Health (Grant Nos. R01MH118695, R01EB020407, and R01MH117107 [to VDC], U01MH111826 [to CCA], and R61MH125126 [to CCA]), the China Natural Science Foundation (Grant No. 61773380 [to JS]), and the Beijing Municipal Science and Technology Commission (Grant No. Z181100001518005 [to JS]).
The authors report no biomedical financial interests or potential conflicts of interest.
Supplementary Material
Download : Download Acrobat PDF file (3MB)
Supplementary Materials.
References
1 Global Burden of Disease Study 2013 Collaborators
Global, regional, and national incidence, prevalence, and years lived with disability for 301 acute and chronic diseases and injuries in 188 countries, 1990-2013: A systematic analysis for the Global Burden of Disease Study 2013
Lancet, 386 (2015), pp. 743-800
Google Scholar2 C. Otte, S.M. Gold, B.W. Penninx, C.M. Pariante, A. Etkin, M. Fava, et al.
Major depressive disorder
Nat Rev Dis Primers, 2 (2016), p. 16065
View Record in ScopusGoogle Scholar3
J.S. Perrin, S. Merz, D.M. Bennett, J. Currie, D.J. Steele, I.C. Reid, C. Schwarzbauer
Electroconvulsive therapy reduces frontal cortical connectivity in severe depressive disorder
Proc Natl Acad Sci U S A, 109 (2012), pp. 5464-5468 View PDF
CrossRefView Record in ScopusGoogle Scholar4
S.H. Lisanby
Electroconvulsive therapy for depression
N Engl J Med, 357 (2007), pp. 1939-1945
View Record in ScopusGoogle Scholar5
J.A. Van Waarde, H.S. Scholte, L.J.B. Van Oudheusden, B. Verwey, D. Denys, G.A. Van Wingen
A functional MRI marker may predict the outcome of electroconvulsive therapy in severe and treatment-resistant depression
Mol Psychiatry, 20 (2015), pp. 609-614 View PDF
CrossRefView Record in ScopusGoogle Scholar6
F. Bouckaert, P. Sienaert, J. Obbels, A. Dols, M. Vandenbulcke, M. Stek, T. Bolwig
ECT: Its brain enabling effects: A review of electroconvulsive therapy-induced structural brain plasticity
J ECT, 30 (2014), pp. 143-151
View Record in ScopusGoogle Scholar7
S. Qi, C.C. Abbott, K.L. Narr, R. Jiang, J. Upston, S.M. McClintock, et al.
Electroconvulsive therapy treatment responsive multimodal brain networks
Hum Brain Mapp, 41 (2020), pp. 1775-1785 View PDF
CrossRefView Record in ScopusGoogle Scholar8
H. Sun, R. Jiang, S. Qi, K.L. Narr, B.S. Wade, J. Upston, et al.
Preliminary prediction of individual response to electroconvulsive therapy using whole-brain functional magnetic resonance imaging data
Neuroimage Clin, 26 (2020), p. 102080
ArticleDownload PDFView Record in ScopusGoogle Scholar9
R. Jiang, C.C. Abbott, T. Jiang, Y. Du, R. Espinoza, K.L. Narr, et al.
SMRI biomarkers predict electroconvulsive treatment outcomes: Accuracy with independent data sets
Neuropsychopharmacology, 43 (2018), pp. 1078-1087 View PDF
CrossRefView Record in ScopusGoogle Scholar10
H.A. Sackeim, J. Prudic, R. Fuller, J. Keilp, P.W. Lavori, M. Olfson
The cognitive effects of electroconvulsive therapy in community settings
Neuropsychopharmacology, 32 (2007), pp. 244-254 View PDF
CrossRefView Record in ScopusGoogle Scholar11
M. Semkovska, D.M. McLoughlin
Objective cognitive performance associated with electroconvulsive therapy for depression: A systematic review and meta-analysis
Biol Psychiatry, 68 (2010), pp. 568-577
ArticleDownload PDFView Record in ScopusGoogle Scholar12
R.D. Weiner, H.J. Rogers, J.R. Davidson, L.R. Squire
Effects of stimulus parameters on cognitive side effects
Ann N Y Acad Sci, 462 (1986), pp. 315-325 View PDF
L.R. Squire
Memory functions as affected by electroconvulsive therapy
Ann N Y Acad Sci, 462 (1986), pp. 307-314 View PDF
CrossRefView Record in ScopusGoogle Scholar14
H.A. Sackeim, J. Prudic, M.S. Nobler, L. Fitzsimons, S.H. Lisanby, N. Payne, et al.
Effects of pulse width and electrode placement on the efficacy and cognitive effects of electroconvulsive therapy
Brain Stimul, 1 (2008), pp. 71-83
ArticleDownload PDFView Record in ScopusGoogle Scholar15
T.D. Perera, B. Luber, M.S. Nobler, J. Prudic, C. Anderson, H.A. Sackeim
Seizure expression during electroconvulsive therapy: Relationships with clinical outcome and cognitive side effects
Neuropsychopharmacology, 29 (2004), pp. 813-825
View Record in ScopusGoogle Scholar16
A. Schat, W.W. Van Den Broek, P.G.H. Mulder, T.K. Birkenhäger, R. Van Tuijl, J.M.J. Murre
Changes in everyday and semantic memory function after electroconvulsive therapy for unipolar depression
J ECT, 23 (2007), pp. 153-157 View PDF
CrossRefView Record in ScopusGoogle Scholar17
A. Fujita, S. Nakaaki, K. Segawa, H. Azuma, K. Sato, K. Arahata, et al.
Memory, attention, and executive functions before and after sine and pulse wave electroconvulsive therapies for treatment-resistant major depression
J ECT, 22 (2006), pp. 107-112 View PDF
CrossRefView Record in ScopusGoogle Scholar18
P.R. Bosboom, J.B. Deijen
Age-related cognitive effects of ECT and ECT-induced mood improvement in depressive patients
Depress Anxiety, 23 (2006), pp. 93-101 View PDF
CrossRefView Record in ScopusGoogle Scholar19
H.A. Fox, A. Rosen, R.J. Campbell
Are brief pulse and sine wave ECT equally efficient?
J Clin Psychiatry, 50 (1989), pp. 432-435
View Record in ScopusGoogle Scholar20
A. Ingram, M.M. Saling, I. Schweitzer
Cognitive side effects of brief pulse electroconvulsive therapy: A review
J ECT, 24 (2008), pp. 3-9
View Record in ScopusGoogle Scholar21
H.A. Sackeim, J. Prudic, D.P. Devanand, M.S. Nobler, S.H. Lisanby, S. Peyser, et al.
A prospective, randomized, double-blind comparison of bilateral and right unilateral electroconvulsive therapy at different stimulus intensities
Arch Gen Psychiatry, 57 (2000), pp. 425-434
View Record in ScopusGoogle Scholar22
J.A. Gray
Brain systems that mediate both emotion and cognition
Cogn Emot, 4 (1990), pp. 269-288 View PDF
CrossRefView Record in ScopusGoogle Scholar23
A. Berger
Self-Regulation: Brain, Cognition, and Development
American Psychological Association, Washington, DC (2011)
H.L. Urry, C.M. Van Reekum, T. Johnstone, N.H. Kalin, M.E. Thurow, H.S. Schaefer, et al.
Amygdala and ventromedial prefrontal cortex are inversely coupled during regulation of negative affect and predict the diurnal pattern of cortisol secretion among older adults
J Neurosci, 26 (2006), pp. 4415-4425
View Record in ScopusGoogle Scholar25
S.C. Matthews, I.A. Strigo, A.N. Simmons, T.T. Yang, M.P. Paulus
Decreased functional coupling of the amygdala and supragenual cingulate is related to increased depression in unmedicated individuals with current major depressive disorder
J Affect Disord, 111 (2008), pp. 13-20
ArticleDownload PDFGoogle Scholar26
C.H. Chen, J. Suckling, C. Ooi, C.H.Y. Fu, S.C.R. Williams, N.D. Walsh, et al.
Functional coupling of the amygdala in depressed patients treated with antidepressant medication
Neuropsychopharmacology, 33 (2008), pp. 1909-1918 View PDF
CrossRefView Record in ScopusGoogle Scholar27
Y.I. Sheline, J.L. Price, Z. Yan, M.A. Mintun
Resting-state functional MRI in depression unmasks increased connectivity between networks via the dorsal nexus
Proc Natl Acad Sci U S A, 107 (2010), pp. 11020-11025 View PDF
CrossRefView Record in ScopusGoogle Scholar28
C.C. Abbott, T. Jones, N.T. Lemke, P. Gallegos, S.M. McClintock, A.R. Mayer, et al.
Hippocampal structural and functional changes associated with electroconvulsive therapy response
Transl Psychiatry, 4 (2014), p. e483 View PDF
CrossRefView Record in ScopusGoogle Scholar29
C.C. Abbott, N.T. Lemke, S. Gopal, R.J. Thoma, J. Bustillo, V.D. Calhoun, J.A. Turner
Electroconvulsive therapy response in major depressive disorder: A pilot functional network connectivity resting state fMRI investigation
Front Psychiatry, 4 (2013), p. 10 View PDF
CrossRefView Record in ScopusGoogle Scholar30
K.J. Mitchell, M.K. Johnson, C.L. Raye, M. D’Esposito
fMRI evidence of age-related hippocampal dysfunction in feature binding in working memory
Brain Res Cogn Brain Res, 10 (2000), pp. 197-206
ArticleDownload PDFView Record in ScopusGoogle Scholar31
B. Rypma, J.S. Berger, H.M. Genova, D. Rebbechi, M. D’Esposito
Dissociating age-related changes in cognitive strategy and neural efficiency using event-related fMRI
Cortex, 41 (2005), pp. 582-594
ArticleDownload PDFView Record in ScopusGoogle Scholar32
N.C. Dvornek, P. Ventola, K.A. Pelphrey, J.S. Duncan
Identifying autism from resting-state fMRI using long short-term memory networks
Mach Learn Med Imaging, 10541 (2017), pp. 362-370 View PDF
CrossRefView Record in ScopusGoogle Scholar33
F. Peters, F. Collette, C. Degueldre, V. Sterpenich, S. Majerus, E. Salmon
The neural correlates of verbal short-term memory in Alzheimer’s disease: An fMRI study
Brain, 132 (2009), pp. 1833-1846 View PDF
CrossRefView Record in ScopusGoogle Scholar34
M.C. Jackson, C. Wolf, S.J. Johnston, J.E. Raymond, D.E.J. Linden
Neural correlates of enhanced visual short-term memory for angry faces: An fMRI study
PLoS One, 3 (2008), Article e3536 View PDF
CrossRefView Record in ScopusGoogle Scholar35
R.M. Hutchison, T. Womelsdorf, E.A. Allen, P.A. Bandettini, V.D. Calhoun, M. Corbetta, et al.
Dynamic functional connectivity: Promise, issues, and interpretations
Neuroimage, 80 (2013), pp. 360-378
ArticleDownload PDFView Record in ScopusGoogle Scholar36
E.A. Allen, E. Damaraju, S.M. Plis, E.B. Erhardt, T. Eichele, V.D. Calhoun
Tracking whole-brain connectivity dynamics in the resting state
Cereb Cortex, 24 (2014), pp. 663-676 View PDF
CrossRefView Record in ScopusGoogle Scholar37
C. Chang, G.H. Glover
Time-frequency dynamics of resting-state brain connectivity measured with fMRI
Neuroimage, 50 (2010), pp. 81-98
ArticleDownload PDFView Record in ScopusGoogle Scholar38
E.A. Allen, E. Damaraju, T. Eichele, L. Wu, V.D. Calhoun
EEG signatures of dynamic functional network connectivity states
Brain Topogr, 31 (2018), pp. 101-116 View PDF
CrossRefView Record in ScopusGoogle Scholar39
X. Liu, J.H. Duyn
Time-varying functional network information extracted from brief instances of spontaneous brain activity
Proc Natl Acad Sci U S A, 110 (2013), pp. 4392-4397 View PDF
CrossRefView Record in ScopusGoogle Scholar40
R.M. Hutchison, T. Womelsdorf, J.S. Gati, S. Everling, R.S. Menon
Resting-state networks show dynamic functional connectivity in awake humans and anesthetized macaques
Hum Brain Mapp, 34 (2013), pp. 2154-2177 View PDF
CrossRefView Record in ScopusGoogle Scholar41
Y. Du, S.L. Fryer, Z. Fu, D. Lin, J. Sui, J. Chen, et al.
Dynamic functional connectivity impairments in early schizophrenia and clinical high-risk for psychosis
Neuroimage, 180 (2018), pp. 632-645
ArticleDownload PDFView Record in ScopusGoogle Scholar42
D. Yao, V.D. Calhoun, Z. Fu, Y. Du, J. Sui
An ensemble learning system for a 4-way classification of Alzheimer’s disease and mild cognitive impairment
J Neurosci Methods, 302 (2018), pp. 75-81
ArticleDownload PDFView Record in ScopusGoogle Scholar43
J. Kim, M. Criaud, S.S. Cho, M. Díez-Cirarda, A. Mihaescu, S. Coakeley, et al.
Abnormal intrinsic brain functional network dynamics in Parkinson’s disease
Brain, 140 (2017), pp. 2955-2967 View PDF
CrossRefView Record in ScopusGoogle Scholar44
Z. Fu, A. Iraji, J.A. Turner, J. Sui, R. Miller, G.D. Pearlson, V.D. Calhoun
Dynamic state with covarying brain activity-connectivity: On the pathophysiology of schizophrenia
Neuroimage, 224 (2021), p. 117385
ArticleDownload PDFView Record in ScopusGoogle Scholar45
Z. Fu, Y. Tu, X. Di, Y. Du, J. Sui, B.B. Biswal, et al.
Transient increased thalamic-sensory connectivity and decreased whole-brain dynamism in autism
Neuroimage, 190 (2019), pp. 191-204
ArticleDownload PDFView Record in ScopusGoogle Scholar46
A. Abrol, E. Damaraju, R.L. Miller, J.M. Stephen, E.D. Claus, A.R. Mayer, V.D. Calhoun
Replicability of time-varying connectivity patterns in large resting state fMRI samples
Neuroimage, 163 (2017), pp. 160-176
ArticleDownload PDFView Record in ScopusGoogle Scholar47
Z. Fu, A. Caprihan, J. Chen, Y. Du, J.C. Adair, J. Sui, et al.
Altered static and dynamic functional network connectivity in Alzheimer’s disease and subcortical ischemic vascular disease: Shared and specific brain connectivity abnormalities
Hum Brain Mapp, 40 (2019), pp. 3203-3221 View PDF
CrossRefView Record in ScopusGoogle Scholar48
Y. Du, Z. Fu, J. Sui, S. Gao, Y. Xing, D. Lin, et al.
NeuroMark: An automated and adaptive ICA based pipeline to identify reproducible fMRI markers of brain disorders
NeuroImage Clin, 28 (2020), p. 102375
ArticleDownload PDFView Record in ScopusGoogle Scholar49
R.L. Miller, M. Yaesoubi, J.A. Turner, D. Mathalon, A. Preda, G. Pearlson, et al.
Higher dimensional meta-state analysis reveals reduced resting fMRI connectivity dynamism in schizophrenia patients
PLoS One, 11 (2016), Article e0149849 View PDF
CrossRefView Record in ScopusGoogle Scholar50
T. Matsui, T. Murakami, K. Ohki
Neuronal origin of the temporal dynamics of spontaneous BOLD activity correlation
Cereb Cortex, 29 (2019), pp. 1496-1508 View PDF
CrossRefView Record in ScopusGoogle Scholar51
Z. Ma, N. Zhang
Temporal transitions of spontaneous brain activity
Elife, 7 (2018), Article e33562
K.N. Gurney, M.D. Humphries, P. Redgrave
A new framework for cortico-striatal plasticity: Behavioural theory meets in vitro data at the reinforcement-action interface
PLoS Biol, 13 (2015), Article e1002034 View PDF
P. Piray, I. Toni, R. Cools
Human choice strategy varies with anatomical projections from ventromedial prefrontal cortex to medial striatum
J Neurosci, 36 (2016), pp. 2857-2867
View Record in ScopusGoogle Scholar54
E. Damaraju, E.A. Allen, A. Belger, J.M. Ford, S. McEwen, D.H. Mathalon, et al.
Dynamic functional connectivity analysis reveals transient states of dysconnectivity in schizophrenia
Neuroimage Clin, 5 (2014), pp. 298-308
ArticleDownload PDFView Record in ScopusGoogle Scholar55
B. Rashid, E. Damaraju, G.D. Pearlson, V.D. Calhoun
Dynamic connectivity states estimated from resting fMRI Identify differences among Schizophrenia, bipolar disorder, and healthy control subjects
Front Hum Neurosci, 8 (2014), p. 897
View Record in ScopusGoogle Scholar56
R.H. Kaiser, J.R. Andrews-Hanna, T.D. Wager, D.A. Pizzagalli
Large-scale network dysfunction in major depressive disorder: A meta-analysis of resting-state functional connectivity
JAMA Psychiatry, 72 (2015), pp. 603-611 View PDF
CrossRefView Record in ScopusGoogle Scholar57
K. Kim, S.W. Kim, W. Myung, C.E. Han, M. Fava, D. Mischoulon, et al.
Reduced orbitofrontal-thalamic functional connectivity related to suicidal ideation in patients with major depressive disorder
Sci Rep, 7 (2017), p. 15772
View Record in ScopusGoogle Scholar58
T.D. Wager, M.L. Davidson, B.L. Hughes, M.A. Lindquist, K.N. Ochsner
Prefrontal-subcortical pathways mediating successful emotion regulation
Neuron, 59 (2008), pp. 1037-1050
ArticleDownload PDFView Record in ScopusGoogle Scholar59
T. Johnstone, C.M. Van Reekum, H.L. Urry, N.H. Kalin, R.J. Davidson
Failure to regulate: Counterproductive recruitment of top-down prefrontal-subcortical circuitry in major depression
J Neurosci, 27 (2007), pp. 8877-8884
View Record in ScopusGoogle Scholar60
M.G. Preti, T.A. Bolton, D. Van De Ville
The dynamic functional connectome: State-of-the-art and perspectives
Neuroimage, 160 (2017), pp. 41-54
ArticleDownload PDFView Record in ScopusGoogle Scholar61
M. Demirtaş, C. Tornador, C. Falcón, M. López-Solà, R. Hernández-Ribas, J. Pujol, et al.
Dynamic functional connectivity reveals altered variability in functional connectivity among patients with major depressive disorder
Hum Brain Mapp, 37 (2016), pp. 2918-2930 View PDF
CrossRefView Record in ScopusGoogle Scholar62
R.H. Kaiser, S. Whitfield-Gabrieli, D.G. Dillon, F. Goer, M. Beltzer, J. Minkel, et al.
Dynamic resting-state functional connectivity in major depression
Neuropsychopharmacology, 41 (2016), pp. 1822-1830 View PDF
CrossRefView Record in ScopusGoogle Scholar63
M. Wei, J. Qin, R. Yan, K. Bi, C. Liu, Z. Yao, Q. Lu
Association of resting-state network dysfunction with their dynamics of inter-network interactions in depression
J Affect Disord, 174 (2015), pp. 527-534
ArticleDownload PDFView Record in ScopusGoogle Scholar64
J.R. Andrews-Hanna, J.S. Reidler, J. Sepulcre, R. Poulin, R.L. Buckner
Functional-anatomic fractionation of the Brain’s default network
Neuron, 65 (2010), pp. 550-562
ArticleDownload PDFView Record in ScopusGoogle Scholar65
D.D. Dougherty, A.P. Weiss, G.R. Cosgrove, N.M. Alpert, E.H. Cassem, A.A. Nierenberg, et al.
Cerebral metabolic correlates as potential predictors of response to anterior cingulotomy for treatment of major depression
J Neurosurg, 99 (2003), pp. 1010-1017 View PDF
CrossRefView Record in ScopusGoogle Scholar66
V.A. Holthoff, B. Beuthien-Baumann, G. Zündorf, A. Triemer, S. Lüdecke, P. Winiecki, et al.
Changes in brain metabolism associated with remission in unipolar major depression
Acta Psychiatr Scand, 110 (2004), pp. 184-194
View Record in ScopusGoogle Scholar67
M.D. Greicius, B.H. Flores, V. Menon, G.H. Glover, H.B. Solvason, H. Kenna, et al.
Resting-state functional connectivity in major depression: Abnormally increased contributions from subgenual cingulate cortex and thalamus
Biol Psychiatry, 62 (2007), pp. 429-437
ArticleDownload PDFView Record in ScopusGoogle Scholar68
L. Schmaal, D.J. Veltman, T.G.M. Van Erp, P.G. Sämann, T. Frodl, N. Jahanshad, et al.
Subcortical brain alterations in major depressive disorder: Findings from the ENIGMA Major Depressive Disorder working group
Mol Psychiatry, 21 (2016), pp. 806-812 View PDF
CrossRefView Record in ScopusGoogle Scholar69
P. Nordanskog, U. Dahlstrand, M.R. Larsson, E.M. Larsson, L. Knutsson, A. Johanson
Increase in hippocampal volume after electroconvulsive therapy in patients with depression: A volumetric magnetic resonance imaging study
J ECT, 26 (2010), pp. 62-67
View Record in ScopusGoogle Scholar70
H.A. Marusak, V.D. Calhoun, S. Brown, L.M. Crespo, K. Sala-Hamrick, I.H. Gotlib, M.E. Thomason
Dynamic functional connectivity of neurocognitive networks in children
Hum Brain Mapp, 38 (2017), pp. 97-108 View PDF
CrossRefView Record in ScopusGoogle Scholar71
R.E. Ingram
Self-focused attention in clinical disorders: Review and a conceptual model
Psychol Bull, 107 (1990), pp. 156-176
View Record in ScopusGoogle Scholar72
N. Mor, J. Winquist
Self-focused attention and negative affect: A meta-analysis
Psychol Bull, 128 (2002), pp. 638-662
T.W. Smith, J. Greenberg
Depression and self-focused attention
Motiv Emot, 5 (1981), pp. 323-331
View Record in ScopusGoogle Scholar74
M. Moreno-Ortega, J. Prudic, S. Rowny, G.H. Patel, A. Kangarlu, S. Lee, et al.
Resting state functional connectivity predictors of treatment response to electroconvulsive therapy in depression
Sci Rep, 9 (2019), p. 5071
View Record in ScopusGoogle Scholar75
J. Wang, Q. Wei, L. Wang, H. Zhang, T. Bai, L. Cheng, et al.
Functional reorganization of intra- and internetwork connectivity in major depressive disorder after electroconvulsive therapy
Hum Brain Mapp, 39 (2018), pp. 1403-1411 View PDF
CrossRefView Record in ScopusGoogle Scholar76
T. Bai, Q. Wei, M. Zu, W. Xie, J. Wang, J. Gong-Jun, et al.
Functional plasticity of the dorsomedial prefrontal cortex in depression reorganized by electroconvulsive therapy: Validation in two independent samples
Hum Brain Mapp, 40 (2019), pp. 465-473 View PDF
CrossRefView Record in ScopusGoogle Scholar77
J. Zhang, H. Tian, J. Li, S. Ji, S. Chen, J. Zhu, et al.
Ketamine plus propofol-electroconvulsive therapy (ECT) transiently improves the antidepressant effects and the associated brain functional alterations in patients with propofol-ECT-resistant depression
Psychiatry Res, 287 (2020), p. 112907
ArticleDownload PDFView Record in ScopusGoogle Scholar78
F. Sambataro, P.A. Thomann, H.M. Nolte, J.H. Hasenkamp, D. Hirjak, K.M. Kubera, et al.
Transdiagnostic modulation of brain networks by electroconvulsive therapy in schizophrenia and major depression
Eur Neuropsychopharmacol, 29 (2019), pp. 925-935
ArticleDownload PDFView Record in ScopusGoogle Scholar79
D. Goldberg
The heterogeneity of “major depression”
World Psychiatry, 10 (2011), pp. 226-228 View PDF
CrossRefView Record in ScopusGoogle Scholar80
J.E. Malberg, A.J. Eisch, E.J. Nestler, R.S. Duman
Chronic antidepressant treatment increases neurogenesis in adult rat hippocampus
J Neurosci, 20 (2000), pp. 9104-9110 View PDF
CrossRefView Record in ScopusGoogle Scholar81
E. Watkins, J.D. Teasdale
Rumination and overgeneral memory in depression: Effects of self-focus and analytic thinking
J Abnorm Psychol, 110 (2001), pp. 353-357
View Record in ScopusGoogle Scholar82
M. Fink
The practice of ECT: Recommendations for treatment, training, and privileging — Background
Convuls Ther, 6 (1990), pp. 82-84
View Record in ScopusGoogle Scholar83
M.C. McElhiney, B.J. Moody, B.L. Steif, J. Prudic, D.P. Devanand, M.S. Nobler, H.A. Sackeim
Autobiographical memory and mood: Effects of electroconvulsive therapy
Neuropsychology, 9 (1995), pp. 501-517
View Record in ScopusGoogle Scholar84
C. Mosti, M. Brook
The cognitive effects of electroconvulsive therapy: A critical review
Psychiatr Ann, 49 (2019), pp. 152-156 View PDF
CrossRefView Record in ScopusGoogle Scholar85
L. Douw, D.G. Wakeman, N. Tanaka, H. Liu, S.M. Stufflebeam
State-dependent variability of dynamic functional connectivity between frontoparietal and default networks relates to cognitive flexibility
Neuroscience, 339 (2016), pp. 12-21
ArticleDownload PDFView Record in ScopusGoogle Scholar86
P. Lin, Y. Yang, J. Jovicich, N. De Pisapia, X. Wang, C.S. Zuo, J.J. Levitt
Static and dynamic posterior cingulate cortex nodal topology of default mode network predicts attention task performance
Brain Imaging Behav, 10 (2016), pp. 212-225 View PDF
CrossRefView Record in ScopusGoogle Scholar87
S. Sadaghiani, J.B. Poline, A. Kleinschmidt, M. D’Esposito
Ongoing dynamics in large-scale functional connectivity predict perception
Proc Natl Acad Sci U S A, 112 (2015), pp. 8463-8468 View PDF
CrossRefView Record in ScopusGoogle Scholar88
J.R. Petrella, S.E. Prince, L. Wang, C. Hellegers, P.M. Doraiswamy
Prognostic value of posteromedial cortex deactivation in mild cognitive impairment
PLoS One, 2 (2007), p. e1104 View PDF
CrossRefView Record in ScopusGoogle Scholar89
C. McCormick, M. Moscovitch, T.A. Valiante, M. Cohn, M.P. McAndrews
Different neural routes to autobiographical memory recall in healthy people and individuals with left medial temporal lobe epilepsy
Neuropsychologia, 110 (2018), pp. 26-36